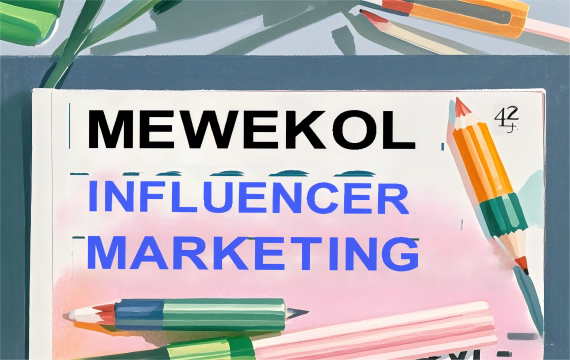
How to Judge the Reliability and Validity of Data Analysis Results?
1. Reliability of Data Sources
-
Scientific Approach to Data Collection
- Evaluate Data Collection Channels: Ensure that data is collected through reliable methods. For instance, when collecting fan data for celebrities, it’s important to verify whether the data is sourced directly from a social media platform’s official interface or gathered via trusted third-party monitoring tools. If data is obtained through a survey, ensure the questionnaire design is unbiased, with clear and neutral questions that avoid leading respondents or sampling bias. For example, when investigating the purchasing intent of celebrity fans, the questions should be direct and neutral, and the sample should represent a diverse range of fan types.
- Completeness of Data Collection: Assess whether all relevant data points have been captured. For example, when evaluating the reach of a celebrity’s content, it's not enough to only look at exposure; you should also examine other metrics like clicks, engagement time, and interactions. Missing key data could lead to inaccurate analysis and unreliable results.
-
Credibility of the Data Provider
- Evaluate the Data Provider: If third-party data is used, assess the credibility of the provider. Reputable data analysis companies with a strong track record and professional methodology are generally more reliable. On the other hand, data from less-known or disreputable sources may be inaccurate or even misleading.
- Verify the Authenticity of the Data Source: Trace the origin of the data to ensure its authenticity. For example, if a data provider claims to have internal data from a social media platform, confirm that the platform has authorized the use of this data. Lack of proper verification could cast doubt on the data’s reliability.
2. Rationality of Data Analysis Methods
-
Applicability of Analysis Methods
- Select the Right Analysis Method: Choose analysis methods that are suited to the type of data and the research question at hand. For instance, time series analysis is ideal for studying the growth trends of celebrity fanbases, while multiple regression analysis is better suited for examining factors affecting the success of celebrity-brand collaborations. Using an inappropriate analysis method, such as employing correlation analysis to establish causal relationships, could lead to erroneous conclusions.
- Check Assumptions: Every data analysis method has its assumptions. For example, linear regression assumes a linear relationship between variables, and residuals must follow a normal distribution. It's important to verify that the data meets these assumptions before proceeding. If the data doesn’t align, it may need to be transformed, or a different method should be chosen.
-
Accuracy of the Analysis Process
- Data Cleaning and Preprocessing: Confirm that the data has been properly cleaned. For example, when analyzing celebrity engagement data, ensure that duplicates, outliers, or irrelevant entries (such as fake likes or comments) have been removed. Inaccurate data cleaning can distort the analysis and lead to misleading results.
- Model Construction and Parameter Estimation: When using statistical models, make sure that the model is constructed correctly and that parameters are estimated using suitable techniques (such as the least squares method). Additionally, check that the model has been tested for accuracy using goodness-of-fit tests or other diagnostic checks to confirm its reliability.
3. Result Verification and Cross-Validation
-
Internal Verification
- Repeat the Analysis: To check the reliability of the results, repeat the analysis using the same dataset and methodology. If the results vary widely each time, it could indicate instability in the analysis process, suggesting unreliable outcomes. For example, when calculating the sales conversion rate of a celebrity’s promotion, the results should fall within a reasonable margin of error. If they differ significantly, you may need to review the data and methods used.
- Use Different Tools or Software: Whenever possible, use different data analysis tools or software to perform the same analysis. For example, compare results obtained from Excel with those from more specialized statistical software like SPSS. This helps identify potential issues with the tools or errors due to user mistakes.
-
External Cross-Validation
- Compare with Industry Reports or Other Studies: Compare the results of your analysis with industry reports or similar studies to see if they align. If your findings significantly diverge from the industry norm or authoritative studies, it’s worth revisiting the analysis process. For instance, if your analysis indicates unusually high fan activity for a celebrity compared to industry benchmarks, it could signal a flaw in the data collection or analysis approach.
- Consult Experts and Real-World Conditions: Consider whether the analysis results match actual market conditions and seek input from experts in the field. For example, if data suggests a celebrity has low commercial value, but industry experts and market trends suggest otherwise, you should re-evaluate your findings and methodology to identify potential errors.