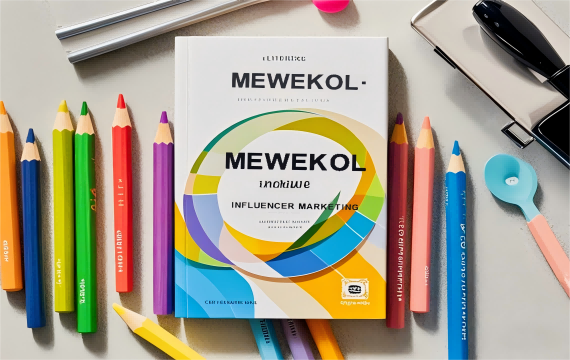
How to Judge Whether Data Analysis Results Align with Business Logic?
1. Evaluate in Combination with Business Goals
-
Clear Business Objectives: To assess the alignment of data analysis with business logic, it's essential to first clarify the core objectives of the influencer marketing campaign. For example, a brand might aim to increase product sales, enhance brand awareness, or expand its customer base. If the data analysis shows that product sales did not increase significantly after influencer promotion but brand awareness grew substantially, this could still be in line with the brand’s broader goal of market expansion. In this case, the brand may have aimed to first increase visibility, with the intention of converting awareness into future sales.
-
Compare Expectations with Actual Results: It’s important to compare the results of data analysis with the business’s pre-set expectations. For instance, if the brand aimed to increase market share by 5% within a month through influencer collaboration, but the actual increase was only 2%, it’s crucial to analyze why this happened. Was the influencer promotion insufficient, or was there increased competition in the market? If the actual results are reasonably close to the expectations and the differences can be explained logically, then the analysis aligns with business logic to a certain extent.
2. Think from the Perspective of Market and Industry Trends
-
Consider Market Trends: Data analysis should be evaluated against broader market trends. For example, in the beauty industry, if data reveals that the audience for a beauty influencer focused on natural ingredients is growing rapidly, it aligns with the rising consumer demand for natural and organic products. Conversely, if data shows that a celebrity’s promotion of a junk food brand led to an unusual sales increase during a time when consumers are prioritizing healthy diets, without any supporting explanation (e.g., promotional campaigns or product improvements), the analysis may not follow business logic.
-
Refer to Industry Standards and Practices: Every industry has its own set of standards and expected outcomes. For instance, in fashion, partnerships between clothing brands and fashion influencers typically result in measurable traffic and sales growth. If data analysis shows no such increase after collaboration with a prominent influencer, it contradicts industry expectations. Further investigation is needed to determine if the lack of impact is due to ineffective promotion methods, unappealing products, or errors in data collection.
3. Judgment Based on Consumer Behavior Theory
-
Analysis of the Consumer Decision-Making Process: According to consumer behavior theory, purchasing decisions usually involve stages such as awareness, interest, evaluation, and purchase. If data analysis reveals that celebrity promotions led to significant awareness but low conversion rates, this might indicate issues at the interest or evaluation stages. Perhaps the product is priced too high or there are doubts about its quality. This result aligns with the typical consumer decision-making process, where awareness doesn’t always translate directly into sales.
-
Consumer Psychological Factors: Consider the psychological factors that influence consumer behavior, such as trust, social proof, and herd mentality. For example, if data analysis shows that consumers are more likely to purchase products recommended by multiple celebrities, this reflects the influence of social proof. However, if the analysis shows that consumers are purchasing products endorsed by a celebrity with low trust and a poor reputation—without any compelling reason, such as an extremely low price—it may contradict typical consumer psychology. In this case, the data's accuracy should be re-evaluated.
4. Observe the Causal Relationship and Correlation between Data
-
Causal Relationship Rationality: Assess whether the causal relationships presented in the data analysis are reasonable. For instance, if the data suggests that an increase in the number of product review videos by influencers directly led to higher product sales, this makes sense from a business perspective. However, if the data shows that more life-record videos unrelated to the product resulted in a sales increase, without a plausible explanation (such as subtle product placements within the videos), this causal relationship does not align with business logic.
-
Practical Significance of Correlation: Examine whether correlations in the data have practical implications for the business. For example, if the geographical distribution of an influencer’s fanbase aligns with the brand’s target market, and sales in those regions increase after the influencer’s promotion, the correlation is meaningful and consistent with business objectives. On the other hand, if the age distribution of an influencer’s fans doesn’t align with the target age group of the product, yet sales increase inexplicably, further investigation is needed to understand the cause. This might require additional analysis to determine whether the results align with the brand's strategic intentions.